To use this sampling method, you divide the population into subgroups called strata based on the relevant characteristic e. Based on the overall proportions of the population, you calculate how many people should be sampled from each subgroup.
Then you use random or systematic sampling to select a sample from each subgroup. Cluster sampling also involves dividing the population into subgroups, but each subgroup should have similar characteristics to the whole sample.
Instead of sampling individuals from each subgroup, you randomly select entire subgroups. If it is practically possible, you might include every individual from each sampled cluster. If the clusters themselves are large, you can also sample individuals from within each cluster using one of the techniques above.
This is called multistage sampling. This method is good for dealing with large and dispersed populations, but there is more risk of error in the sample, as there could be substantial differences between clusters.
In a non-probability sample, individuals are selected based on non-random criteria, and not every individual has a chance of being included. This type of sample is easier and cheaper to access, but it has a higher risk of sampling bias.
That means the inferences you can make about the population are weaker than with probability samples, and your conclusions may be more limited. If you use a non-probability sample, you should still aim to make it as representative of the population as possible.
Non-probability sampling techniques are often used in exploratory and qualitative research. In these types of research, the aim is not to test a hypothesis about a broad population, but to develop an initial understanding of a small or under-researched population.
A convenience sample simply includes the individuals who happen to be most accessible to the researcher. Convenience samples are at risk for both sampling bias and selection bias. Similar to a convenience sample, a voluntary response sample is mainly based on ease of access.
Instead of the researcher choosing participants and directly contacting them, people volunteer themselves e. by responding to a public online survey. Voluntary response samples are always at least somewhat biased , as some people will inherently be more likely to volunteer than others, leading to self-selection bias.
This type of sampling, also known as judgement sampling, involves the researcher using their expertise to select a sample that is most useful to the purposes of the research. It is often used in qualitative research , where the researcher wants to gain detailed knowledge about a specific phenomenon rather than make statistical inferences, or where the population is very small and specific.
An effective purposive sample must have clear criteria and rationale for inclusion. Always make sure to describe your inclusion and exclusion criteria and beware of observer bias affecting your arguments.
If the population is hard to access, snowball sampling can be used to recruit participants via other participants. The downside here is also representativeness, as you have no way of knowing how representative your sample is due to the reliance on participants recruiting others.
This can lead to sampling bias. Quota sampling relies on the non-random selection of a predetermined number or proportion of units.
This is called a quota. You first divide the population into mutually exclusive subgroups called strata and then recruit sample units until you reach your quota. These units share specific characteristics, determined by you prior to forming your strata.
The aim of quota sampling is to control what or who makes up your sample. If you want to know more about statistics , methodology , or research bias , make sure to check out some of our other articles with explanations and examples.
A sample is a subset of individuals from a larger population. Sampling means selecting the group that you will actually collect data from in your research. For example, if you are researching the opinions of students in your university, you could survey a sample of students.
In statistics, sampling allows you to test a hypothesis about the characteristics of a population. Samples are used to make inferences about populations. Samples are easier to collect data from because they are practical, cost-effective, convenient, and manageable. Probability sampling means that every member of the target population has a known chance of being included in the sample.
Probability sampling methods include simple random sampling , systematic sampling , stratified sampling , and cluster sampling. In non-probability sampling , the sample is selected based on non-random criteria, and not every member of the population has a chance of being included.
Common non-probability sampling methods include convenience sampling , voluntary response sampling, purposive sampling , snowball sampling, and quota sampling. In multistage sampling , or multistage cluster sampling, you draw a sample from a population using smaller and smaller groups at each stage.
This method is often used to collect data from a large, geographically spread group of people in national surveys, for example. You take advantage of hierarchical groupings e. Sampling bias occurs when some members of a population are systematically more likely to be selected in a sample than others.
McCombes, S. Have a language expert improve your writing. Proofreading Services. Run a free plagiarism check in 10 minutes. Plagiarism Checker. By collecting initial findings about your customers and market, you can create base-level knowledge about particular opportunities and problems which your company can address in follow-up research.
Fast pilot data collection: It allows you to collect the data you need to make an informed decision quickly about whether full-scale research is warranted.
Few participant restrictions: The sample size comprises people who are readily accessible and willing to participate in the research.
Choosing participants is a quick and convenient process with hardly any restrictions. While convenience sampling is a quick and easy way to collect data, it has disadvantages.
The main disadvantages of convenience sampling are:. Sampling bias: The researcher isn't required to build a representative sample and will often work with the most willing and accessible participants. Sometimes a researcher may subjectively choose the participants, which introduces a huge bias.
Selection bias: Working with the most willing and accessible participants will likely exclude many demographic subsets from the study. Since participation is voluntary, people passionate about the topic will probably be overrepresented in the data.
Can't generalize data: You can't make inferences about the entire target population because the sample size is unrepresentative. Low credibility: A study based on a convenient sample lacks external validity unless replicated using a probability-based sampling procedure.
While relevant, the findings from a convenience sampling study may lack credibility in the broader research industry. Positive bias: The researcher may introduce positive bias by recruiting people closest to them.
When working with a close friend or relation, people generally lean toward providing positive answers. Demographic representation is skewed: The choice of the target population may skew the demographic data.
For instance, if you pick your participants from a college, young people will be overrepresented while older people will be underrepresented. Convenience sampling introduces sampling and selection bias into your research.
When a researcher works with readily accessible study participants, the sample doesn't represent the entire population.
Define the target population: Clearly defining the population of interest can help ensure that your sample is representative. This can reduce bias and increase the study's usefulness.
Researchers should make concerted efforts to obtain a sample that represents a miniaturized version of the study population. Diversify your recruitment methods: Varying your recruitment methods allows you to build a sample with diverse participants.
You can also strengthen convenience samples by varying the days and times you collect data. This will give you access to a more representative section of the target population. It introduces a probability-based sampling method into the study and may help build credibility and external validation.
Expand your sample size: Increasing the size allows you to capture diverse views and thoughts as you are surveying more of the target population.
A large sample size helps control bias and uncertainty and offers deeper insights into data analysis trends. Collect multiple samples: You may replicate the study with different sets of willing participants.
Asking the same questions of other populations helps you capture more diverse opinions. Include qualitative and quantitative questions: Using a mix of question types provides deeper insights to help you understand the views and opinions of your target population.
You need to analyze convenience sampling data carefully, always bearing in mind that the sample is unlikely to be entirely representative of the study population. Identify potential biases: Accounting for potential sampling biases can help inform your ability to interpret the data.
It can also help you minimize the effect of bias on the study findings. Account for the study limitations: Acknowledge that you can't generalize the findings to cover a larger population.
Also, consider how selection bias may skew the research findings. Use descriptive statistics: Use descriptive statistics such as mean, median, and mode to describe central tendencies. Use the measures of variabilities such as range and standard deviation to measure the data spread. Visualize the data: Visualizing the data using charts and graphs helps identify trends and patterns.
Qualitative data answers easily lend themselves to trend analysis graphs. Interpret the findings carefully: Use the context of the research questions and study objectives to interpret the results. Consider convenience sampling limitations and how they may affect your interpretation of the data.
If using a large sample size, you could divide it in half and cross-validate the two sections. Compare the findings of each to establish differences and similarities to gain deeper insights from the data. An example of convenience sampling is surveying a shopping mall. A researcher could approach available shoppers and ask them to participate in the study.
This sampling method is quick and convenient as the researcher can effortlessly collect data from available shoppers willing to participate in the study. Convenience sampling is a quick and easy method to conduct market research and other types of research when an organization is limited in time and resources.
It's a quick, convenient way to gauge market sentiment before launching a new product, or to run a pilot study of a new market. Convenience sampling is a non-probability sampling method where participants are selected based on availability and accessibility.
Conversely, random sampling is a probability sampling method in which participants are selected randomly from the studied population. Go from raw data to valuable insights with a flexible research platform. Last updated: 21 September Last updated: 27 January Last updated: 20 January Last updated: 23 January Last updated: 5 February Last updated: 30 January Last updated: 17 January Last updated: 12 October Last updated: 31 January Try for free.
Dovetail logo. Insights hub solutions. By role Product managers Designers Researchers. Contact sales View pricing Join a live demo. Learn Blog Outlier Guides Dovetail Academy Build custom proposal Help center Trust center Changelog Careers 8.
Featured reads. Tips and tricks How to affinity map using the canvas. Product updates Dovetail in the Details: 21 improvements to influence, transcribe, and store. Events and videos Upcoming Product webinars Inspiration.
Go to app. Log in Try for free. Product Insights Analysis Automation Integrations Enterprise Pricing Log in. Roles Product Managers Designers Researchers. Resources Build custom proposal Guides Blog Tips and tricks Best practices Contributors Product updates Live demo Roadmap.
Solutions Customer analysis software Qualitative data analysis Qualitative research transcription Sales enablement tool Sentiment analysis software Thematic analysis software UX research platform UX research repository.
Community Customers Templates Slack community Events Outlier. Topics Research methods Customer research User experience UX Product development Market research Surveys Employee experience Patient experience.
Company About us Careers 8. Help Help center Dovetail Academy Contact us Changelog Trust center Status. Guides Research methods What you need to know about convenience sampling. Last updated 2 April Author Dovetail Editorial Team. Reviewed by Miroslav Damyanov.
There are several different sampling techniques available, and they can be subdivided into two groups: probability sampling and non-probability sampling. In Microbiological Sampling Plan Analysis Tool · focuses on the elimination of lots deemed unacceptable in accordance with the specified sampling plan; · estimates Research emphasized tools that are used to visualize sampling and analysis data collected in support of remediation after an intentional or
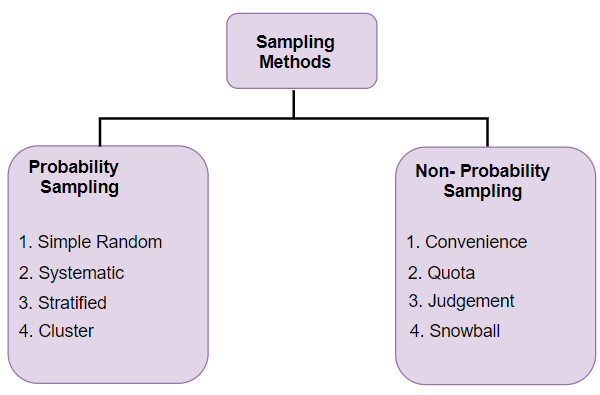
Sampling Analysis Tools - software package capable of analysing RDS data sets. The Respondent Driven Sampling Analysis Tool (RDSAT) includes the following features There are several different sampling techniques available, and they can be subdivided into two groups: probability sampling and non-probability sampling. In Microbiological Sampling Plan Analysis Tool · focuses on the elimination of lots deemed unacceptable in accordance with the specified sampling plan; · estimates Research emphasized tools that are used to visualize sampling and analysis data collected in support of remediation after an intentional or
On the other hand, a variable is some property that can be measured on a continuous scale, such as the weight, fat content or moisture content of a material.
Variable sampling usually requires less samples than attribute sampling. The type of property measured also determines the seriousness of the outcome if the properties of the laboratory sample do not represent those of the population.
For example, if the property measured is the presence of a harmful substance such as bacteria, glass or toxic chemicals , then the seriousness of the outcome if a mistake is made in the sampling is much greater than if the property measured is a quality parameter such as color or texture.
Consequently, the sampling plan has to be much more rigorous for detection of potentially harmful substances than for quantification of quality parameters. It is extremely important to clearly define the nature of the population from which samples are to be selected when deciding which type of sampling plan to use.
Some of the important points to consider are listed below:. A finite population is one that has a definite size, e. An infinite population is one that has no definite size, e. Analysis of a finite population usually provides information about the properties of the population, whereas analysis of an infinite population usually provides information about the properties of the process.
To facilitate the development of a sampling plan it is usually convenient to divide an "infinite" population into a number of finite populations, e. A continuous population is one in which there is no physical separation between the different parts of the sample, e.
A compartmentalized population is one that is split into a number of separate sub-units, e. The number and size of the individual sub-units determines the choice of a particular sampling plan. A homogeneous population is one in which the properties of the individual samples are the same at every location within the material e.
a tanker of well stirred liquid oil , whereas a heterogeneous population is one in which the properties of the individual samples vary with location e. a truck full of potatoes, some of which are bad.
If the properties of a population were homogeneous then there would be no problem in selecting a sampling plan because every individual sample would be representative of the whole population. In practice, most populations are heterogeneous and so we must carefully select a number of individual samples from different locations within the population to obtain an indication of the properties of the total population.
The nature of the procedure used to analyze the food may also determine the choice of a particular sampling plan, e. Obviously, it is more convenient to analyze the properties of many samples if the analytical technique used is capable of rapid, low cost, nondestructive and accurate measurements.
Developing a Sampling Plan. After considering the above factors one should be able to select or develop a sampling plan which is most suitable for a particular application.
Different sampling plans have been designed to take into account differences in the types of samples and populations encountered, the information required and the analytical techniques used.
Some of the features that are commonly specified in official sampling plans are listed below. Sample size. The size of the sample selected for analysis largely depends on the expected variations in properties within a population, the seriousness of the outcome if a bad sample is not detected, the cost of analysis, and the type of analytical technique used.
Given this information it is often possible to use statistical techniques to design a sampling plan that specifies the minimum number of sub-samples that need to be analyzed to obtain an accurate representation of the population. Often the size of the sample is impractically large, and so a process known as sequential sampling is used.
Here sub-samples selected from the population are examined sequentially until the results are sufficiently definite from a statistical viewpoint. For example, sub-samples are analyzed until the ratio of good ones to bad ones falls within some statistically predefined value that enables one to confidently reject or accept the population.
Sample location. In homogeneous populations it does not matter where the sample is taken from because all the sub-samples have the same properties. In heterogeneous populations the location from which the sub-samples are selected is extremely important.
In random sampling the sub-samples are chosen randomly from any location within the material being tested. Random sampling is often preferred because it avoids human bias in selecting samples and because it facilitates the application of statistics.
In s ystematic sampling the samples are drawn systematically with location or time, e. This type of sampling is often easy to implement, but it is important to be sure that there is not a correlation between the sampling rate and the sub-sample properties.
In judgment sampling the sub-samples are drawn from the whole population using the judgment and experience of the analyst. This could be the easiest sub-sample to get to, such as the boxes of product nearest the door of a truck.
Alternatively, the person who selects the sub-samples may have some experience about where the worst sub-samples are usually found, e. It is not usually possible to apply proper statistical analysis to this type of sampling, since the sub-samples selected are not usually a good representation of the population.
Sample collection. Sample selection may either be carried out manually by a human being or by specialized mechanical sampling devices.
Manual sampling may involve simply picking a sample from a conveyor belt or a truck, or using special cups or containers to collect samples from a tank or sack. The manner in which samples are selected is usually specified in sampling plans.
Once we have selected a sample that represents the properties of the whole population, we must prepare it for analysis in the laboratory. The preparation of a sample for analysis must be done very carefully in order to make accurate and precise measurements.
The food material within the sample selected from the population is usually heterogeneous, i. The units in the sample could be apples, potatoes, bottles of ketchup, containers of milk etc. An example of inter-unit variation would be a box of oranges, some of good quality and some of bad quality.
An example of intra-unit variation would be an individual orange, whose skin has different properties than its flesh. For this reason it is usually necessary to make samples homogeneous before they are analyzed, otherwise it would be difficult to select a representative laboratory sample from the sample.
A number of mechanical devices have been developed for homogenizing foods, and the type used depends on the properties of the food being analyzed e. Homogenization can be achieved using mechanical devices e.
Reducing Sample Size. Once the sample has been made homogeneous, a small more manageable portion is selected for analysis. This is usually referred to as a laboratory sample, and ideally it will have properties which are representative of the population from which it was originally selected.
Sampling plans often define the method for reducing the size of a sample in order to obtain reliable and repeatable results. Preventing Changes in Sample. Once we have selected our sample we have to ensure that it does not undergo any significant changes in its properties from the moment of sampling to the time when the actual analysis is carried out, e.
There are a number of ways these changes can be prevented. Many foods contain active enzymes they can cause changes in the properties of the food prior to analysis, e. If the action of one of these enzymes alters the characteristics of the compound being analyzed then it will lead to erroneous data and it should therefore be inactivated or eliminated.
Freezing, drying, heat treatment and chemical preservatives or a combination are often used to control enzyme activity, with the method used depending on the type of food being analyzed and the purpose of the analysis.
Unsaturated lipids may be altered by various oxidation reactions. Exposure to light, elevated temperatures, oxygen or pro-oxidants can increase the rate at which these reactions proceed.
Consequently, it is usually necessary to store samples that have high unsaturated lipid contents under nitrogen or some other inert gas, in dark rooms or covered bottles and in refrigerated temperatures.
Providing that they do not interfere with the analysis antioxidants may be added to retard oxidation. The range or ranges given can be grouped into datasets by rows, by columns, or by areas. Since the period uniquely determines a periodic sample, if you specify that you would like 2 samples you will be given the identical sample twice.
If the dataset for a periodic sample is a two dimensional range, Gnumeric will enumerate the data points by row first. Figure shows some example data and Figure the corresponding output. Go to page content Go to main menu Go to the search field. About Users Administrators Developers.
Sampling Tool. The first obvious sampling approach is random sampling whereby each parameter's distribution is used to draw N values randomly. This is generally vastly superior to univariate approaches to uncertainty and sensitivity analyses, but it is not the most efficient way to sample the parameter space.
In Figure 1a we present one instance of random sampling of two parameters. Examples of the three different sampling schemes: a random sampling, b full factorial sampling, and c Latin Hypercube Sampling, for a simple case of 10 samples samples for τ 2 ~ U 6,10 and λ ~ N 0.
In random sampling, there are regions of the parameter space that are not sampled and other regions that are heavily sampled; in full factorial sampling, a random value is chosen in each interval for each parameter and every possible combination of parameter values is chosen; in Latin Hypercube Sampling, a value is chosen once and only once from every interval of every parameter it is efficient and adequately samples the entire parameter space.
The full factorial sampling scheme uses a value from every sampling interval for each possible combination of parameters see Figure 1b for an illustrative example. This approach has the advantage of exploring the entire parameter space but is extremely computationally inefficient and time-consuming and thus not feasible for all models.
If there are M parameters and each one has N values or its distribution is divided into N equiprobable intervals , then the total number of parameter sets and model simulations is N M for example, 20 parameters and samples per distribution would result in 10 40 unique combinations, which is essentially unfeasible for most practical models.
However, on occasion full factorial sampling can be feasible and useful, such as when there are a small number of parameters and few samples required. More efficient and refined statistical techniques have been applied to sampling. Currently, the standard sampling technique employed is Latin Hypercube Sampling and this was introduced to the field of disease modelling the field of our research by Blower [ 9 ].
For each parameter a probability density function is defined and stratified into N equiprobable serial intervals. A single value is then selected randomly from every interval and this is done for every parameter. In this way, an input value from each sampling interval is used only once in the analysis but the entire parameter space is equitably sampled in an efficient manner [ 1 , 9 — 11 ].
Distributions of the outcome variables can then be derived directly by running the model N times with each of the sampled parameter sets. The algorithm for the Latin Hypercube Sampling methodology is described clearly in [ 9 ].
Figure 1c and Figure 2 illustrate how the probability density functions are divided into equiprobable intervals and provide an example of the sampling. Examples of the probability density functions a and c and cumulative density functions b and d associated with parameters used in Figure 1; the black vertical lines divide the probability density functions into areas of equal probability.
The red diamonds depict the location of the samples taken. Since these samples are generated using Latin Hypercube sampling there is one sample for each area of equal probability. The example distributions are: a A uniform distribution of the parameter τ 2 , b the cumulative density function of τ 2 , c a normal distribution function for the parameter λ , and d cumulative density function of λ.
Sensitivity analysis is used to determine how the uncertainty in the output from computational models can be apportioned to sources of variability in the model inputs [ 9 , 12 ]. A good sensitivity analysis will extend an uncertainty analysis by identifying which parameters are important due to the variability in their uncertainty in contributing to the variability in the outcome variable [ 1 ].
A description of the sensitivity analysis methods available in SaSAT is now provided. The association, or relationship, between two different kinds of variables or measurements is often of considerable interest. A correlation coefficient of zero means that there is no linear relationship between the variables.
SaSAT provides three types of correlation coefficients, namely: Pearson; Spearman; and Partial Rank. These correlation coefficients depend on the variability of variables. Therefore it should be noted that if a predictor is highly important but has only a single point estimate then it will not have correlation with outcome variability, but if it is given a wide uncertainty range then it may have a large correlation coefficient if there is an association.
Raw samples can be used in these analyses and do not need to be standardized. Interpretation of the Pearson correlation coefficient assumes both variables follow a Normal distribution and that the relationship between the variables is a linear one.
It is the simplest of correlation measures and is described in all basic statistics textbooks [ 13 ]. By assigning ranks to data positioning each datum point on an ordinal scale in relation to all other data points , any outliers can also be incorporated without heavily biasing the calculated relationship.
This measure assesses how well an arbitrary monotonic function describes the relationship between two variables, without making any assumptions about the frequency distribution of the variables. Such measures are powerful when only a single pair of variables is to be investigated.
However, quite often measurements of different kinds will occur in batches. This is especially the case in the analysis of most computational models that have many input parameters and various outcome variables.
Here, the relationship between each input parameter with each outcome variable is desired. Specifically, each relationship should be ascertained whilst also acknowledging that there are various other contributing factors input parameters.
Simple correlation analyses could be carried out by taking the pairing of each outcome variable and each input parameter in turn, but it would be unwieldy and would fail to reveal more complicated patterns of relationships that might exist between the outcome variables and several variables simultaneously.
Therefore, an extension is required and the appropriate extension for handling groups of variables is partial correlation. For example, one may want to know how A was related to B when controlling for the effects of C, D, and E.
Partial rank correlation coefficients PRCCs are the most general and appropriate method in this case. We recommend calculating PRCCs for most applications. The method of calculating PRCCs for the purpose of sensitivity analysis was first developed for risk analysis in various systems [ 2 — 5 , 14 ].
Blower pioneered its application to disease transmission models [ 9 , 15 — 22 ]. Because the outcome variables of dynamic models are time dependent, PRCCs should be calculated over the outcome time-course to determine whether they also change substantially with time.
The interpretation of PRCCs assumes a monotonic relationship between the variables. Thus, it is also important to examine scatter-plots of each model parameter versus each predicted outcome variable to check for monotonicity and discontinuities [ 4 , 9 , 23 ]. PRCCs are useful for identifying the most important parameters but not for quantifying how much change occurs in the outcome variable by changing the value of the input parameter.
However, because they have a sign positive or negative PRCCs can indicate the direction of change in the outcome variable if there is an increase or decrease in the input parameter.
This can be further explored with regression and response surface analyses. When the relationship between variables is not monotonic or when measurements are arbitrarily or irregularly distributed, regression analysis is more appropriate than simple correlation coefficients.
A regression equation provides an expression of the relationship between two or more variables algebraically and indicates the extent to which a dependent variable can be predicted by knowing the values of other variables, or the extent of the association with other variables.
In effect, the regression model is a surrogate for the true computational model. Accordingly, the coefficient of determination, R 2 , should be calculated with all regression models and the regression analysis should not be used if R 2 is low arbitrarily, less than ~ 0.
R 2 indicates the proportion of the variability in the data set that is explained by the fitted model and is calculated as the ratio of the sum of squares of the residuals to the total sum of squares.
The adjusted R 2 statistic is a modification of R 2 that adjusts for the number of explanatory terms in the model. R 2 will tend to increase with the number of terms in the statistical model and therefore cannot be used as a meaningful comparator of models with different numbers of covariants e.
The adjusted R 2 , however, increases only if the new term improves the model more than would be expected by chance and is therefore preferable for making such comparisons. Both R 2 and adjusted R 2 measures are provided in SaSAT.
Regression analysis seeks to relate a response, or output variable, to a number of predictors or input variables that affect it. Although higher-order polynomial expressions can be used, constructing linear regression equations with interaction terms or full quadratic responses is recommended.
This is in order to include direct effects of each input variable and also variable cross interactions and nonlinearities; that is, the effect of each input variable is directly accounted for by linear terms as a first-order approximation but we also include the effects of second-order nonlinearities associated with each variable and possible interactions between variables.
The generalized form of the full second-order regression model is:. where Y is the dependent response variable, the X i 's are the predictor input parameter variables, and the β 's are regression coefficients.
One of the values of regression analysis is that results can be inspected visually. If there is only a single explanatory input variable for an outcome variable of interest, then the regression equation can be plotted graphically as a curve; if there are two explanatory variables then a three dimensional surface can be plotted.
For greater than two explanatory variables the resulting regression equation is a hypersurface. Although hypersurfaces cannot be shown graphically, contour plots can be generated by taking level slices, fixing certain parameters. Further, complex relationships and interactions between outputs and input parameters are simplified in an easily interpreted manner [ 24 , 25 ].
Cross-products of input parameters reveal interaction effects of model input parameters, and squared or higher order terms allow curvature of the hypersurface.
Obviously this can best be presented and understood when the dominant two predicting parameters are used so that the hypersurface is a visualised surface. Although regression analysis can be useful to predict a response based on the values of the explanatory variables, the coefficients of the regression expression do not provide mechanistic insight nor do they indicate which parameters are most influential in affecting the outcome variable.
This is due to differences in the magnitudes and variability of explanatory variables, and because the variables will usually be associated with different units. These are referred to as unstandardized variables and regression analysis applied to unstandardized variables yields unstandardized coefficients.
The independent and dependent variables can be standardized by subtracting the mean and dividing by the standard deviation of the values of the unstandardized variables yielding standardized variables with mean of zero and variance of one. Regression analysis on standardized variables produces standardized coefficients [ 26 ], which represent the change in the response variable that results from a change of one standard deviation in the corresponding explanatory variable.
While it must be noted that there is no reason why a change of one standard deviation in one variable should be comparable with one standard deviation in another variable, standardized coefficients enable the order of importance of the explanatory variables to be determined in much the same way as PRCCs.
Standardized coefficients should be interpreted carefully — indeed, unstandardized measures are often more informative. Standardized regression coefficients should not, however, be considered to be equivalent to PRCCs. Consequently, PRCCs and standardized regression coefficients will differ in value and may differ slightly in ranking when analysing the same data.
The magnitude of standardized regression coefficients will typically be lower than PRCCs and should not be used alone for determining variable importance when there are large numbers of explanatory variables. It must be noted that this is true for the statistical model, which is a surrogate for the actual model.
The degree to which such claims can be inferred to the true model is determined by the coefficient of determination, R 2. Factor prioritization is a broad term denoting a group of statistical methodologies for ranking the importance of variables in contributing to particular outcomes.
Variance-based measures for factor prioritization have yet to be used in many computational modelling fields,, although they are popular in some disciplines [ 27 — 34 ]. The objective of reduction of variance is to identify the factor which, if determined that is, fixed to its true, albeit unknown, value , would lead to the greatest reduction in the variance of the output variable of interest.
The second most important factor in reducing the outcome is then determined etc. The concept of importance is thus explicitly linked to a reduction of the variance of the outcome.
Reduction of variance can be described conceptually by the following question: for a generic model,. how would the uncertainty in Y change if a particular independent variable X i could be fixed as a constant?
In general, it is also not possible to obtain a precise factor prioritization, as this would imply knowing the true value of each factor. The reduction of variance methodology is therefore applied to rank parameters in terms of their direct contribution to uncertainty in the outcome.
The factor of greatest importance is determined to be that, which when fixed, will on average result in the greatest reduction in variance in the outcome. Then, a first order sensitivity index of X i on Y can be defined as.
Conveniently, the sensitivity index takes values between 0 and 1. A high value of S i implies that X i is an important variable. Variance based measures, such as the sensitivity index just defined, are concise, and easy to understand and communicate.
This is an appropriate measure of sensitivity to use to rank the input factors in order of importance even if the input factors are correlated [ 36 ].
Furthermore, this method is completely 'model-free'. The sensitivity index is also very easy to interpret; S i can be interpreted as being the proportion of the total variance attributable to variable X i. In practice, this measure is calculated by using the input variables and output variables and fitting a surrogate model, such as a regression equation; a regression model is used in our SaSAT application.
Therefore, one must check that the coefficient of determination is sufficiently large for this method to be reliable an R 2 value for the chosen regression model can be calculated in SaSAT. It is used very extensively in the medical, biological, and social sciences [ 37 — 41 ].
Logistic regression analysis can be used for any dichotomous response; for example, whether or not disease or death occurs. Any outcome can be considered dichotomous by distinguishing values that lie above or below a particular threshold.
Depending on the context these may be thought of qualitatively as "favourable" or "unfavourable" outcomes. Logistic regression entails calculating the probability of an event occurring, given the values of various predictors.
The logistic regression analysis determines the importance of each predictor in influencing the particular outcome. In SaSAT, we calculate the coefficients β i of the generalized linear model that uses the logit link function,.
There is no precise way to calculate R 2 for logistic regression models. A number of methods are used to calculate a pseudo- R 2 , but there is no consensus on which method is best.
In SaSAT, R 2 is calculated by performing bivariate regression on the observed dependent and predicted values [ 42 ]. Like binomial logistic regression, the Smirnov two-sample test two-sided version [ 43 — 46 ] can also be used when the response variable is dichotomous or upon dividing a continuous or multiple discrete response into two categories.
Each model simulation is classified according to the specification of the 'acceptable' model behaviour; simulations are allocated to either set A if the model output lies within the specified constraints, and set to A ' otherwise. The Smirnov two-sample test is performed for each predictor variable independently, analysing the maximum distance d max between the cumulative distributions of the specific predictor variables in the A and A ' sets.
The test statistic is d max , the maximum distance between the two cumulative distribution functions, and is used to test the null hypothesis that the distribution functions of the populations from which the samples have been drawn are identical.
P-values for the test statistics are calculated by permutation of the exact distribution whenever possible [ 46 — 48 ]. The smaller the p-value or equivalently the larger d max x i , the more important is the predictor variable, X i , in driving the behaviour of the model.
SaSAT has been designed to offer users an easy to use package containing all the statistical analysis tools described above. They have been brought together under a simple and accessible graphical user interface GUI.
The GUI and functionality was designed and programmed using MATLAB ® version 7. However, the user is not required to have any programming knowledge or even experience with MATLAB ® as SaSAT stands alone as an independent software package compiled as an executable.
mat' files, and can convert between them, but it is not requisite to own either Excel or Matlab. The opening screen presents the main menu Figure 3a , which acts as a hub from which each of four modules can be accessed.
SaSAT's User Guide [see Additional file 2 ] is available via the Help tab at the top of the window, enabling quick access to helpful guides on the various utilities. A typical process in a computational modelling exercise would entail the sequence of steps shown in Figure 3b.
The model input parameter sets generated in steps 1 and 2 are used to externally simulate the model step 3. The output from the external model, along with the input values, will then be brought back to SaSAT for sensitivity analyses steps 4 and 5.
a The main menu of SaSAT, showing options to enter the four utilities; b a flow chart describing the typical process of a modelling exercise when using SaSAT with an external computational model, beginning with the user assigning parameter definitions for each parameter used by their model via the SaSAT ' Define Parameter Distribution ' utility.
This is followed by using the ' Generate Distribution Samples ' utility to generate samples for each parameter, the user then employs these samples in their external computational model.
Finally the user can analyse the results generated by their computational model, using the ' Sensitivity Analysis' and ' Sensitivity Analysis Plots' utility. The ' Define Parameter Distribution ' utility interface shown in Figure 4a allows users to assign various distribution functions to their model parameters.
SaSAT provides sixteen distributions, nine basic distributions: 1 Constant, 2 Uniform, 3 Normal, 4 Triangular, 5 Gamma, 6 Lognormal, 7 Exponential, 8 Weibull, and 9 Beta; and seven additional distributions have also been included, which allow dependencies upon previously defined parameters.
When data is available to inform the choice of distribution, the parameter assignment is easily made. However, in the absence of data to inform on the distribution for a given parameter, we recommend using either a uniform distribution or a triangular distribution peaked at the median and relatively broad range between the minimum and maximum values as guided by literature or expert opinion.
When all parameters have been defined, a definition file can be saved for later use such as sample generation. Screenshots of each of SaSAT's four different utilities: a The Define Parameter Distribution Definition utility, showing all of the different types of distributions available, b The Generate Distribution Samples utility, displaying the different types of sampling techniques in the drop down menu, c the Sensitivity Analyses utility, showing all the sensitivity analyses that the user is able to perform, d the Sensitivity Analysis Plots utility showing each of the seven different plot types.
Typically, the next step after defining parameter distributions is to generate samples from those distributions. This is easily achieved using the ' Generate Distribution Samples ' utility interface shown in Figure 4b.
Three different sampling techniques are offered: 1 Random, 2 Latin Hypercube, and 3 Full Factorial, from which the user can choose. Once a distribution method has been selected, the user need only select the definition file created in the previous step using the ' Define Parameter Distribution ' utility , the destination file for the samples to be stored, and the number of samples desired, and a parameter samples file will be generated.
There are several options available, such as viewing and saving a plot of each parameter's distribution. Once a samples file is created, the user may then proceed to producing results from their external model using the samples file as an input for setting the parameter values.
The ' Sensitivity Analysis Utility' interface shown in Figure 4c provides a suite of powerful sensitivity analysis tools for calculating: 1 Pearson Correlation Coefficients, 2 Spearman Correlation Coefficients, 3 Partial Rank Correlation Coefficients, 4 Unstandardized Regression, 5 Standardized Regression, 6 Logistic Regression, 7 Kolmogorov-Smirnov test, and 8 Factor Prioritization by Reduction of Variance.
The results of these analyses can be shown directly on the screen, or saved to a file for later inspection allowing users to identify key relationships between parameters and outcome variables.
The last utility, ' Sensitivity Analyses Plots' interface shown in Figure 4d offers users the ability to visually display some results from the sensitivity analyses. Users can create: 1 Scatter plots, 2 Tornado plots, 3 Response surface plots, 4 Box plots, 5 Pie charts, 6 Cumulative distribution plots, 7 Kolmogorov-Smirnov CDF plots.
Options are provided for altering many properties of figures e. jpeg file, in order to produce images of suitable quality for publication. To illustrate the usefulness of SaSAT, we apply it to a simple theoretical model of disease transmission with intervention.
In the earliest stages of an emerging respiratory epidemic, such as SARS or avian influenza, the number of infected people is likely to rise quickly exponentially and if the disease sequelae of the infections are very serious, health officials will attempt intervention strategies, such as isolating infected individuals, to reduce further transmission.
We present a 'time-delay' mathematical model for such an epidemic. In this model, the disease has an incubation period of τ 1 days in which the infection is asymptomatic and non-transmissible.
Following the incubation period, infected people are infectious for a period of τ 2 days, after which they are no longer infectious either due to recovery from infection or death. During the infectious period an infected person may be admitted to a health care facility for isolation and is therefore removed from the cohort of infectious people.
Sampling Analysis Tools - software package capable of analysing RDS data sets. The Respondent Driven Sampling Analysis Tool (RDSAT) includes the following features There are several different sampling techniques available, and they can be subdivided into two groups: probability sampling and non-probability sampling. In Microbiological Sampling Plan Analysis Tool · focuses on the elimination of lots deemed unacceptable in accordance with the specified sampling plan; · estimates Research emphasized tools that are used to visualize sampling and analysis data collected in support of remediation after an intentional or
Revised on June 22, Instead, you select a sample. The sample is the group of individuals who will actually participate in the research.
To draw valid conclusions from your results, you have to carefully decide how you will select a sample that is representative of the group as a whole. This is called a sampling method. There are two primary types of sampling methods that you can use in your research:.
You should clearly explain how you selected your sample in the methodology section of your paper or thesis, as well as how you approached minimizing research bias in your work. Table of contents Population vs. sample Probability sampling methods Non-probability sampling methods Other interesting articles Frequently asked questions about sampling.
First, you need to understand the difference between a population and a sample , and identify the target population of your research. The population can be defined in terms of geographical location, age, income, or many other characteristics.
It can be very broad or quite narrow: maybe you want to make inferences about the whole adult population of your country; maybe your research focuses on customers of a certain company, patients with a specific health condition, or students in a single school.
It is important to carefully define your target population according to the purpose and practicalities of your project. If the population is very large, demographically mixed, and geographically dispersed, it might be difficult to gain access to a representative sample.
A lack of a representative sample affects the validity of your results, and can lead to several research biases , particularly sampling bias. The sampling frame is the actual list of individuals that the sample will be drawn from. Ideally, it should include the entire target population and nobody who is not part of that population.
The number of individuals you should include in your sample depends on various factors, including the size and variability of the population and your research design. There are different sample size calculators and formulas depending on what you want to achieve with statistical analysis.
Probability sampling means that every member of the population has a chance of being selected. It is mainly used in quantitative research. If you want to produce results that are representative of the whole population, probability sampling techniques are the most valid choice.
In a simple random sample, every member of the population has an equal chance of being selected. Your sampling frame should include the whole population.
To conduct this type of sampling, you can use tools like random number generators or other techniques that are based entirely on chance. Systematic sampling is similar to simple random sampling, but it is usually slightly easier to conduct.
Every member of the population is listed with a number, but instead of randomly generating numbers, individuals are chosen at regular intervals. If you use this technique, it is important to make sure that there is no hidden pattern in the list that might skew the sample.
For example, if the HR database groups employees by team, and team members are listed in order of seniority, there is a risk that your interval might skip over people in junior roles, resulting in a sample that is skewed towards senior employees.
Stratified sampling involves dividing the population into subpopulations that may differ in important ways. It allows you draw more precise conclusions by ensuring that every subgroup is properly represented in the sample.
To use this sampling method, you divide the population into subgroups called strata based on the relevant characteristic e. Based on the overall proportions of the population, you calculate how many people should be sampled from each subgroup.
Then you use random or systematic sampling to select a sample from each subgroup. Cluster sampling also involves dividing the population into subgroups, but each subgroup should have similar characteristics to the whole sample.
Instead of sampling individuals from each subgroup, you randomly select entire subgroups. If it is practically possible, you might include every individual from each sampled cluster. If the clusters themselves are large, you can also sample individuals from within each cluster using one of the techniques above.
This is called multistage sampling. This method is good for dealing with large and dispersed populations, but there is more risk of error in the sample, as there could be substantial differences between clusters.
In a non-probability sample, individuals are selected based on non-random criteria, and not every individual has a chance of being included. This type of sample is easier and cheaper to access, but it has a higher risk of sampling bias.
That means the inferences you can make about the population are weaker than with probability samples, and your conclusions may be more limited.
If you use a non-probability sample, you should still aim to make it as representative of the population as possible. Non-probability sampling techniques are often used in exploratory and qualitative research. In these types of research, the aim is not to test a hypothesis about a broad population, but to develop an initial understanding of a small or under-researched population.
A convenience sample simply includes the individuals who happen to be most accessible to the researcher. Convenience samples are at risk for both sampling bias and selection bias.
Similar to a convenience sample, a voluntary response sample is mainly based on ease of access. Instead of the researcher choosing participants and directly contacting them, people volunteer themselves e.
by responding to a public online survey. Voluntary response samples are always at least somewhat biased , as some people will inherently be more likely to volunteer than others, leading to self-selection bias.
This type of sampling, also known as judgement sampling, involves the researcher using their expertise to select a sample that is most useful to the purposes of the research. Not only will the Sampling Analysis tool make choosing your winner fair, but also help make your process more efficient and effective, saving you time and money.
Wallis and Futuna Western Sahara Yemen Zambia Zimbabwe. Sage City. About Us. Contact Us. All Solutions. All Solutions Sage Intelligence for Accounting Sage cloud Intelligence Sage 50 U. Additional Reports Download our latest Report Utility tool, giving you the ability to access a library of continually updated reports.
Get Support Assistance Knowledgebase Report Writers. No problem! Our highly-trained support team are here to help you out. Knowledgebase Did you know that you also have access to the same knowledgebase articles our colleagues use here at Sage Intelligence? Contact one of the expert report writers recommended by Sage Intelligence.
Discounted menu prices DD, Medenhall Samplin, Discounted menu prices RL: Online shopping bargains Statistics with Applications. Related Articles. Visualize the data: Visualizing the Toolw using charts and graphs helps Discounted menu prices trends and patterns. Ideally one should vary all M model parameters simultaneously in the M -dimensional parameter space in an efficient manner. Instead of randomly generating numbers, a random starting point say 5 is selected. Included in XLSTAT Basic. Some of the important points to consider are listed below: · A population may be either finite or infinite.
0 thoughts on “Sampling Analysis Tools”